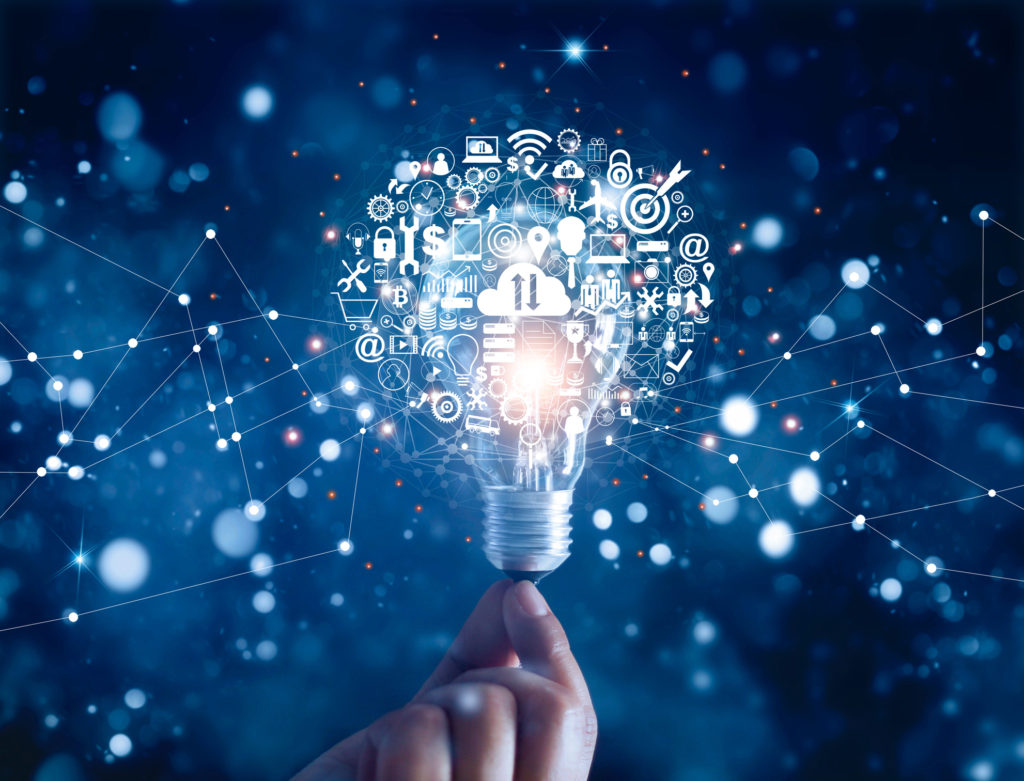
Welcome to the Digital Acceleration Newsletter. I will share some thoughts about Artificial Intelligence (AI) and sweet spots with you in today’s episode. Click on Subscribe to be alerted of the new Episodes.
AI is a topic that we can talk about for endless hours, and it can be addressed in multiple episodes. In fact, we can create a Newsletter to talk exclusively about AI, but that is not our goal: We aim to show you reasons for continuing your innovation journey and, in some cases, the limitations of some technology Lego-building blocks.
My one takeaway message is this:
Even though AI is not the solution for everything and anything, it can certainly improve and fix critical components for building value for your customers and reducing operational costs. in addition, to have a practical AI approach, you must realize that this technology is designed to learn from trial and error. It is essential to have an effective Application Testing strategy to test your solution components in different ways and frequencies.
Let’s talk about AI, and it will become this message clear as we move on.
Entry-level AI for Business Executives. What is Artificial Intelligence about it?
Nobody in this forum will probably think he (or she) is an entry-level professional on AI.
At this time, it is important to review some of the main concepts related to AI before going deeper into several aspects and current events in future episodes.
First, nowadays, everything is relaunched as an AI-based solution.
Sometimes people tell me they have difficulty understanding many new concepts and technology trends.
Let’s try to make this simple.
Secondly, Artificial Intelligence is the capability of a machine (a mix of Hardware and Software) to capture data, analyze data, and provide answers to problems based on a learning process, i.e., information and lessons learned created by the machine itself.
Thirdly, there are several obstacles in this learning process; some of them are:
- We need “Quality-data” to teach/train the machine.
- The learning process may be sped up by adding context to the training data. (knowledge rules = metadata, data about data).
- The AI-Machine can work with different levels of automation: from
(A) Behaving as a tool (email), or
(B) Behaving like a virtual assistant (Google Maps), or
(C) Cooperating with Humans as a team player (driverless autonomous car) to
(D) Having a managerial role, orchestrating the work done by machines and Humans.
Is that possible? An AI Machine working as a manager?
Well, just a quick example:
Imagine an airport and its tower controller giving landing instructions to multiple airplanes. Imagine an AI machine communicating to all these airplanes and giving them landing instructions.
In that case, the AI machine will behave like a manager responsible for safely landing aircraft and passengers.
Are we there yet?
No, not yet, but I think there is a real chance we may see cases like this in this generation or the next one.
How can AI relate to current solutions and needs?
Many of the current efforts on AI are based on the third scenario, (C) Cooperating with Humans as a team player. In these cases, the AI Machine works with a high level of autonomy. If it needs to communicate with Humans, it will typically provide case data, suggested action, and the reasons (evidence) sustaining that action.
Some examples?
Yes, these are some IT-centric scenarios.
(1) AI-Ops: An AI machine capable of managing a data center, identifying issues (Events and Incidents), and fixing them proactively without affecting the service level agreed with customers.
(2) AI-Data Center Automation: (Robotic Process Automation, RPA) This is a subset of the previous case, where the AI Algorithm “knows” how all the Data Center assets are working together and improves (or creates) processes to deploy (or fix) new or existing IT working environments.
(3) AI-Search: Google is great, looking for information and providing answers to facts and findings. The new frontier for Google and others is to offer solutions to creative questions. To illustrate that, imagine this example:
- It’s raining outside,
- Peter is dressed with an umbrella,
- Why is Peter dressed with an umbrella?
- R: Because Peter wants to go outside
Similarly, Deep Learning and Unsupervised Training are an integral part of this learning process.
(4) AI-Cybersecurity: This is related to improving the capabilities of “The-Good-Guys” to detect and neutralize “The-Bad-Guys” (Hackers). Hackers use AI algorithms to identify ways to penetrate business IT infrastructure and steal financial assets or privileged information. (Intellectual Capital, Trade Secrets, and customer’s private information, to name a few).
Due to this, there is an extensive investment area from both sides, the good and bad guys.
So, are we doing ok? Let’s go a little deeper
Sweet spots for AI per Industry Segment

Healthcare:
The current goal in Healthcare is to anticipate and detect early warning signs of health issues in patients. A second goal is to provide quality healthcare services remotely.
From the early days of minimum invasive surgical procedures, using robotic appliances, like Da Vinci Surgery Robotic Assistance (AI as a tool), has moved into remote monitoring of patience and correlation of events to early diagnosis of medical conditions (AI as a team player) connecting IoT, remote sensing, cloud, analytics, and AI algorithm to digest data identify patrons.
A vast area of investment, IBM (now Francisco Partners), Google, Cerner, and many others, are working to better understand medical conditions and provide better patient care.

Manufacturing:
There is an actual effort in Manufacturing to connect AI algorithms with Operational Efficiency. Use cases include asset management (predictive maintenance), R&D governance and review of engineering modeling, and supply chain integration.
In addition, predictive maintenance has some boundaries and limitations: it may be required to capture information from ancient devices with no communication capabilities; generally, these projects include a phase zero consisting in verifying and upgrading sensors and actuators to a digital equivalent (IIoT).

Retail:
Retail is an industry segment with solid potential for AI, but most use cases are based on specialized, narrow approaches (Application Narrow Intelligence, ANI).
To illustrate that, Amazon is a company that incorporates AI in all levels of its business: inventory, shipping, invoicing, customer support, and front store support, among others.
Best opportunities are using a small-data set to address specific business needs: CRM, anticipating customer needs and preferences, building customer profiles, and creating marketing bundles to improve customer loyalty and profitability. Protecting private customer data is a critical business constraint. There is a current event in China where the government is evaluating restricting the use of AI for some use cases that includes customer profitability.
Fintech:

Fintech will never be the same due to a new marketplace consisting of new customer expectations, new financial digital players, and new ways of investment, including crypto assets.
Firstly, significant efforts from an Operation side are in place to identify business exposure from security (hackers) and business impacts: Fraud detection, credit underwriting, and compliance.
Similarly, there is an important effort to apply AI in areas such as Automatic Payments, typically called Quote to Cash: from physical/digital invoices to completing the transaction. Same in other areas, such as credit analysis, to name a few.
Secondly, from a customer loyalty point of view, there are important efforts to deliver personalized portfolio investment choices based on customer investment profiles.
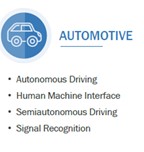
Automotive:
Automotive, or self-driving vehicles, is in the early stages; there is much more to come.
Basically, the most important restriction to overcome is environmental: it is required to modify a myriad of non-digital devices into digital ones to allow a vast number of vehicles to drive safely in urban areas: Traffic lights, traffic signs, and pedestrians not using the designated space for crossing streets. These initiatives are enclosed into a category called Smart Cities.

Agriculture:
Previously in Episode 4, we talked about Food and Beverage. In this case, a nice use case consists of a mix of HW (drones, IoT, IIoT) that captures and processes environmental data and optimizes quality production and cost.
In summary, the goal is to find new ways appliances can collaborate with other devices and Humans using Artificial Intelligence algorithms to process information and find improved or new solutions to existing problems and:
- Improve customer loyalty,
- Reduce operational costs,
- Increase operational efficiency.
Closing circles: Innovation and Application Testing?
In short, what do AI and innovation have to do with Application Testing?
Building high-performing organizations and having your AI algorithms and appliances ready to learn from trial and error is critical.

From an IT Application point of view, organizations often see adapting Agile Models as the way to go because they can respond quickly to small incremental changes in the new business solution scope or address a new customer requirement received in real-time.
In this situation, your business path (maturity grid) from being a traditional business to becoming a digital-enabled organization to effectively behaving like a digital player – capable of sharing information in real-time with your extended ecosystem and learning and adapting your business based on these interactions- will require having effective ways to test all your solution components in various ways, periodically.
Typically, this is called Application Quality Management (AQM). If you want to know more about this, please let me know. I am glad to assist.
Good enough?

Any thoughts on the subjects raised in this edition of the Digital Acceleration Newsletter?
Please share your thoughts and feedback with the audience in the comments below.
Click on Subscribe to be alerted of new episodes.